Abstract:
Image deblurring in photon-limited conditions is ubiquitous in a variety of low-light applications such as photography, microscopy and astronomy. However, the presence of the photon shot noise due to the low illumination and/or short exposure makes the deblurring task substantially more challenging than the conventional deblurring problems. In this paper, we present an algorithm unrolling approach for the photon-limited deblurring problem by unrolling a Plug-and-Play algorithm for a fixed number of iterations. By introducing a three-operator splitting formation of the Plug-and-Play framework, we obtain a series of differentiable steps which allows the fixed iteration unrolled network to be trained end-to-end. The proposed algorithm demonstrates significantly better image recovery compared to existing state-of-the-art deblurring approaches. We also present a new photon-limited deblurring dataset for evaluating the performance of algorithms.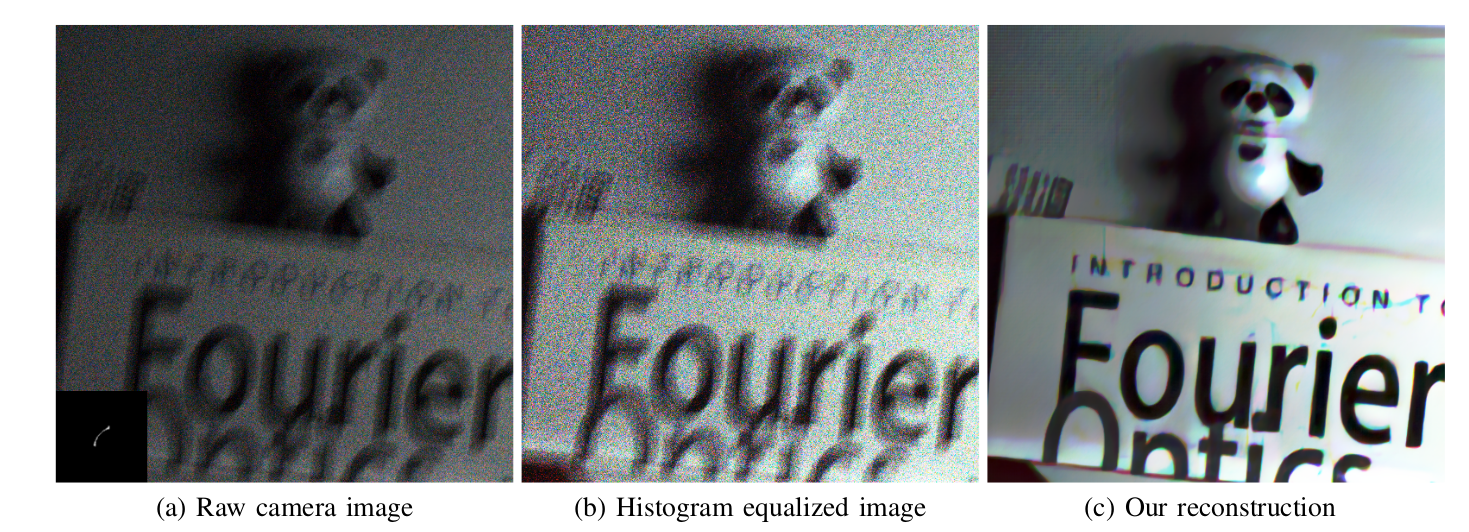
Unrolled Network Architecture using 3-operator Plug-and-Play
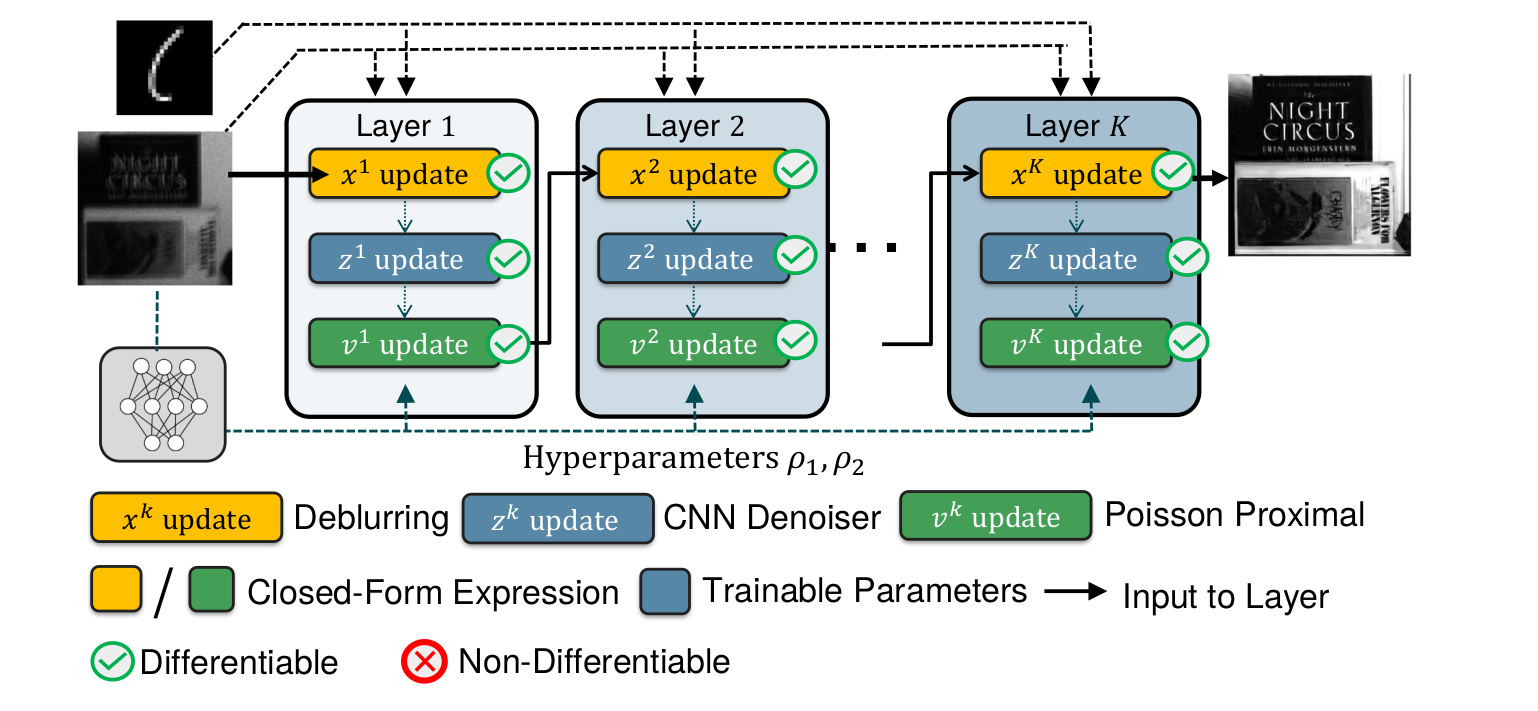
Pretrained model here: OneDrive Google-Drive
ICASSP Video Presentation
Photon-Limited Deblurring Dataset
Real world dataset for evaluation of non-blind deblurring algorithms in the presence of photon shot noise. Contains 30 images at different light levels and blurred by different motion kernels - ground truth kernel captured using a point source.
Feel free to ask your questions/share your feedback at ysanghvi@purdue.edu